Vulnerable road users (VRUs) make up a large proportion of annual road deaths. In 2015, 22% of road fatalities worldwide were pedestrians and 4% were cyclists (World Health Organization, 2015). As the level of motorization increases worldwide, making the interaction between VRUs and motorized vehicles safer is particularly important. While current assistance systems already actively intervene in safety-critical situations (Bengler et al., 2014), with automatic emergency braking and corrective steering interventions for lane keeping, this protective effect can potentially be further enhanced by an effective driver warning system. By informing people early, they can be put in a position to master even complex situations when operating (automated) systems and solve complex problems (Bainbridge, 1983). A timely and targeted warning for the automobile’s driver should enable them to take appropriate accident-avoiding action in good time, even in complex situations.
Many existing driver warning systems already use simple visual (e.g., red flashing light) and auditory features (e.g., high-pitched short sine tones). However, the amount of information and its presentation still needs to be fully utilized. More intelligent processing of the information available from today’s environmental sensor systems may improve the driver warning and better control the driver’s attention at an early stage.
As part of our student project at TUM, we worked with the Environment Perception Team of Daimler AG in Ulm to develop, integrate, and evaluate a multimodal warning concept to warn drivers of dangers posed by or for VRUs. Design recommendations from the literature and previous projects, such as the warning kit developed in the EU project UR:BAN (Bengler, Drüke, Hoffmann, Manstetten & Neukum, 2018), served as a starting point. As part of the project, a human-machine interface (HMI) was created to display three warning levels (early warning, warning, and acute warning), focusing on visual and auditory signals.
We realized the developed concept as a working prototype. We implemented it in an actual vehicle of Daimler AG to qualitatively evaluate the HMI with prospective users and realistic data of the environment-detecting sensor system. In particular, the prototype combines a fully controllable LED strip in the vehicle cockpit with auditory icons as signal sounds. These components are intended to provide the driver with more information about the type of critical situation, considering the classification of the object (e.g., pedestrian), its position, and the criticality of the scenario. We needed to limit the evaluation to pedestrian scenarios for time constraints and safety reasons. In addition to a structured assessment of the concept and the prototype, optimization potential and improvement measures for further development were derived from the evaluation.
Research & Analysis
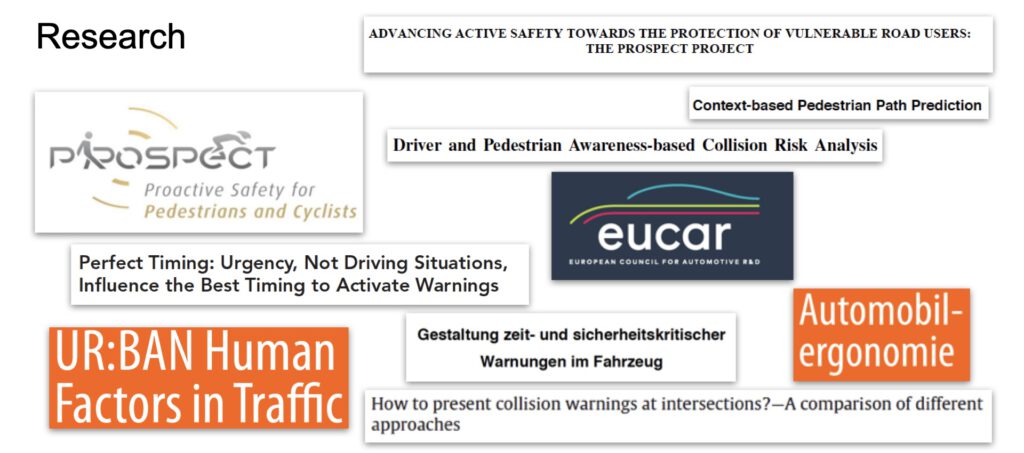
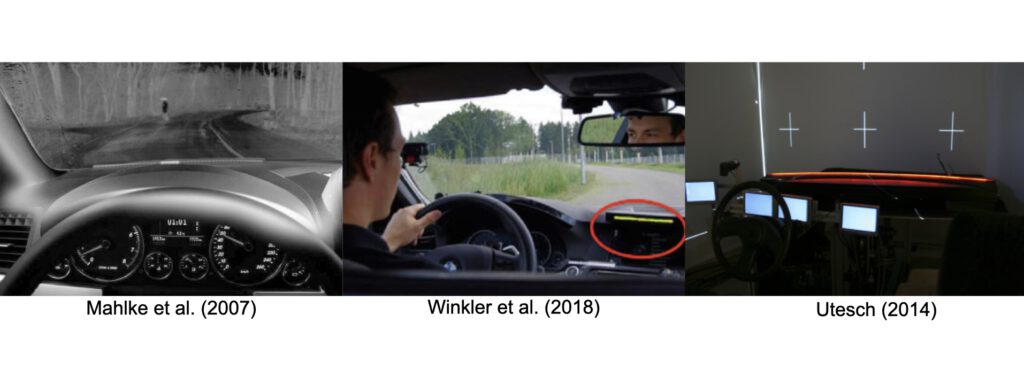
Concept Development
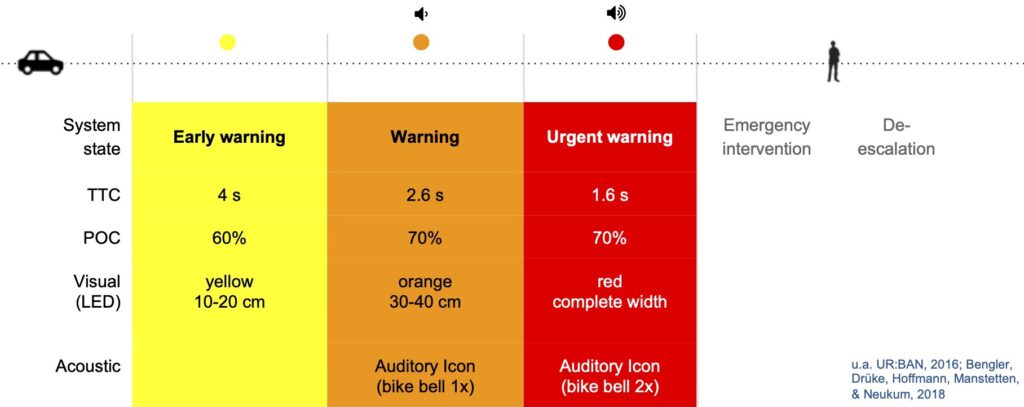
Visual warning
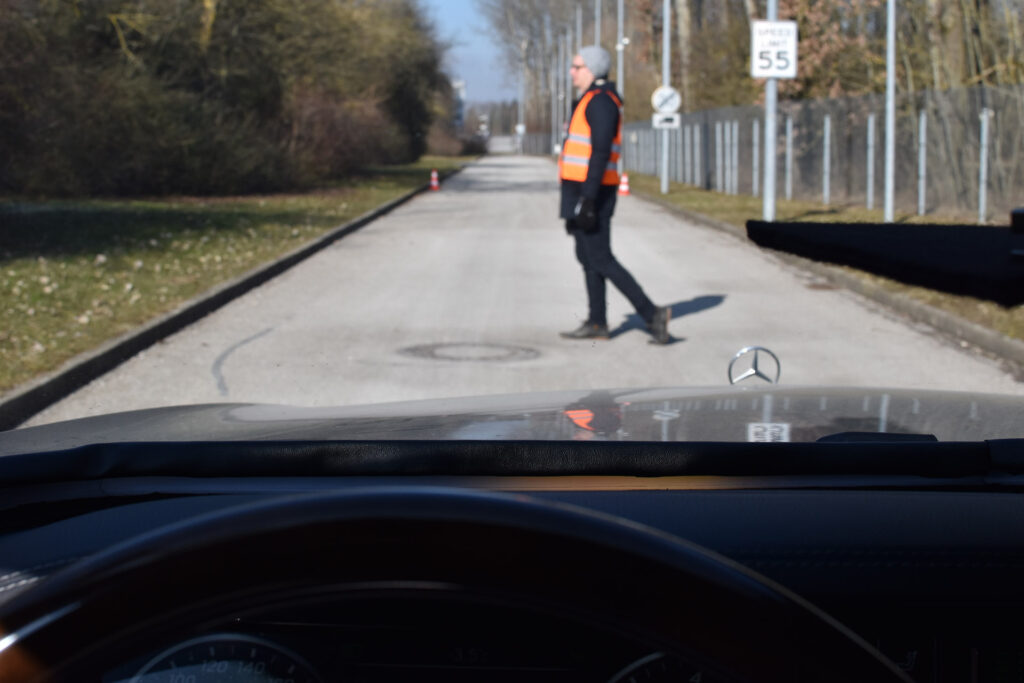
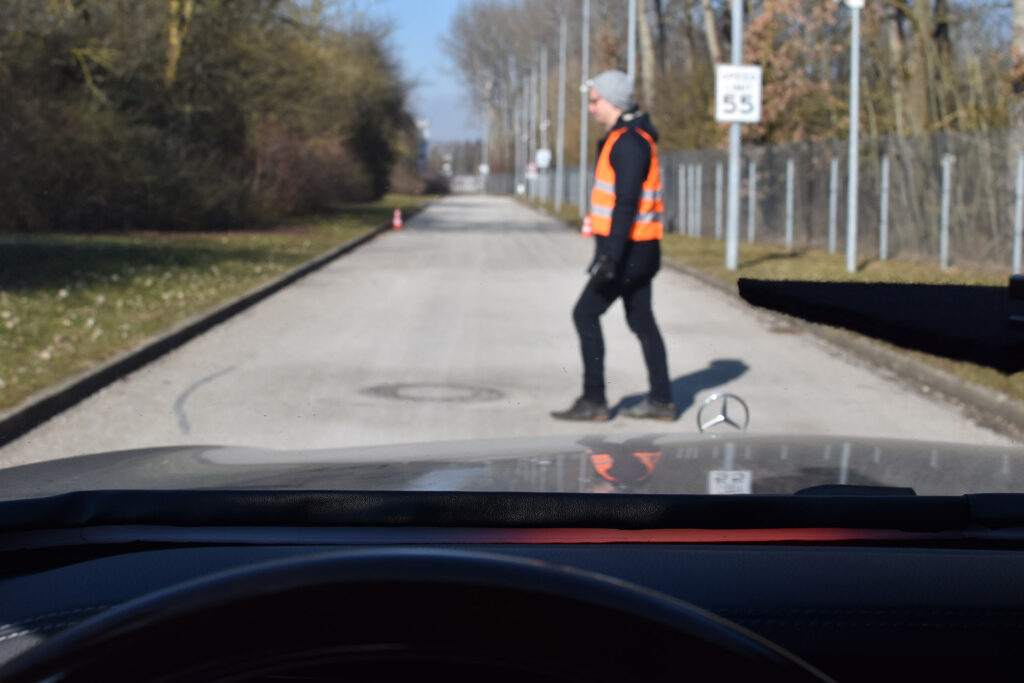
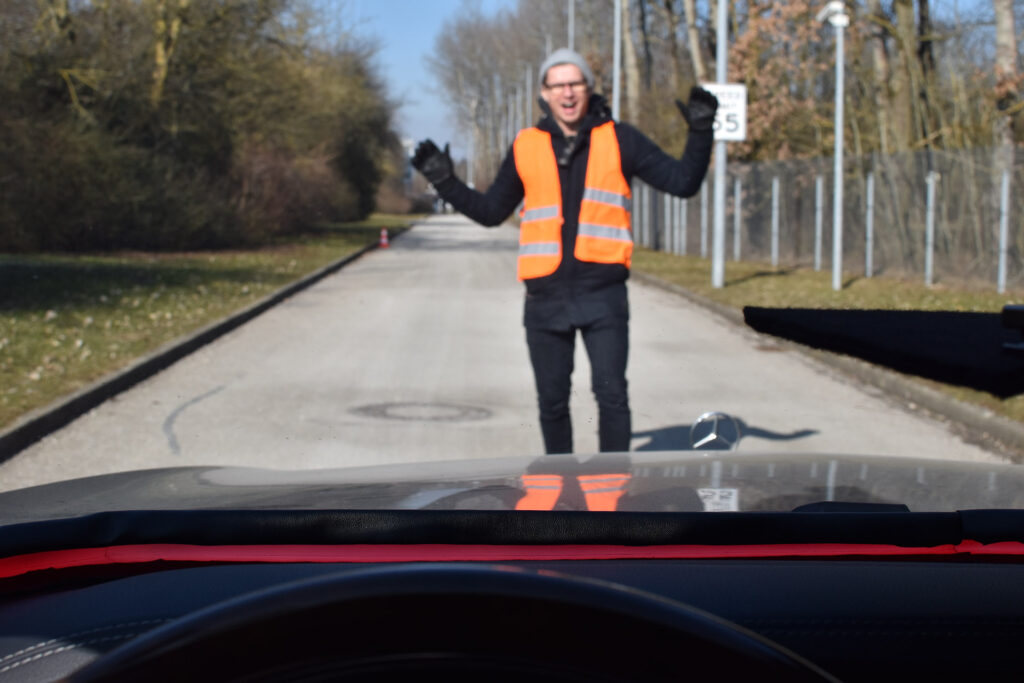
Prototyping & Development Challenges
Hardware: brightness, reflections, inclination of windshield, mounting of components.
Software: communication vehicle & prototype, C++/Arduino coding, data transfer rates, driver problems.
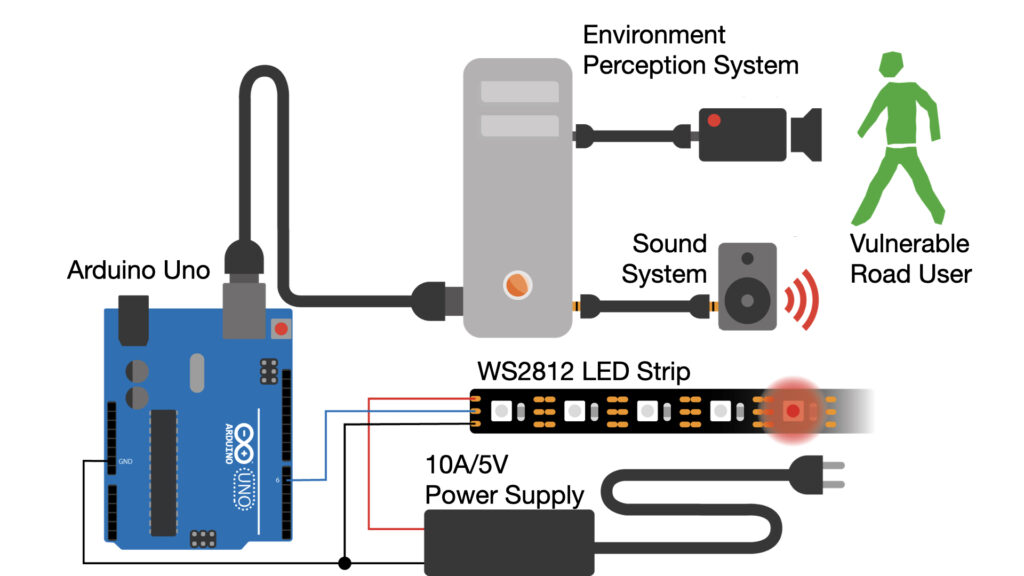
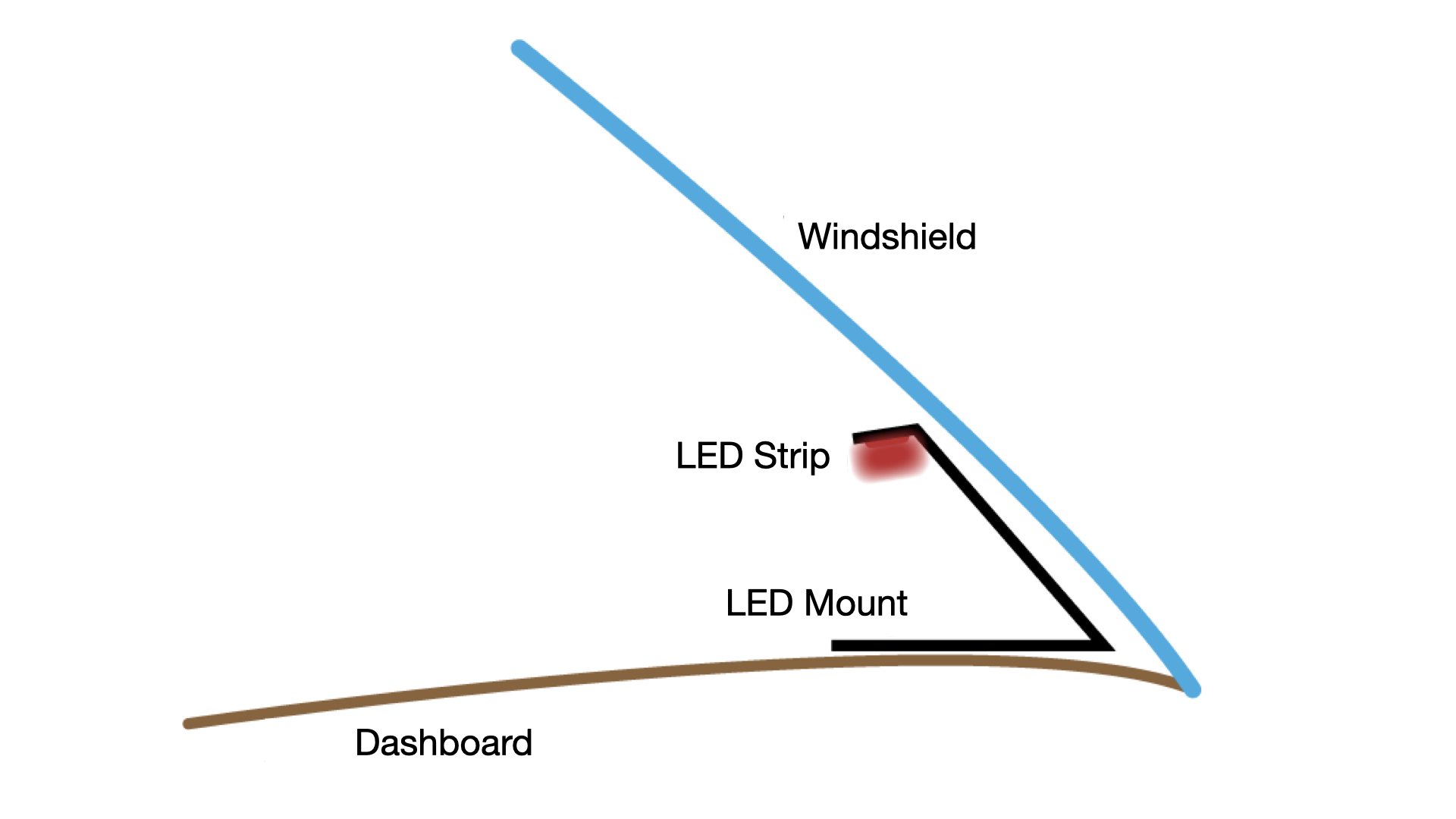
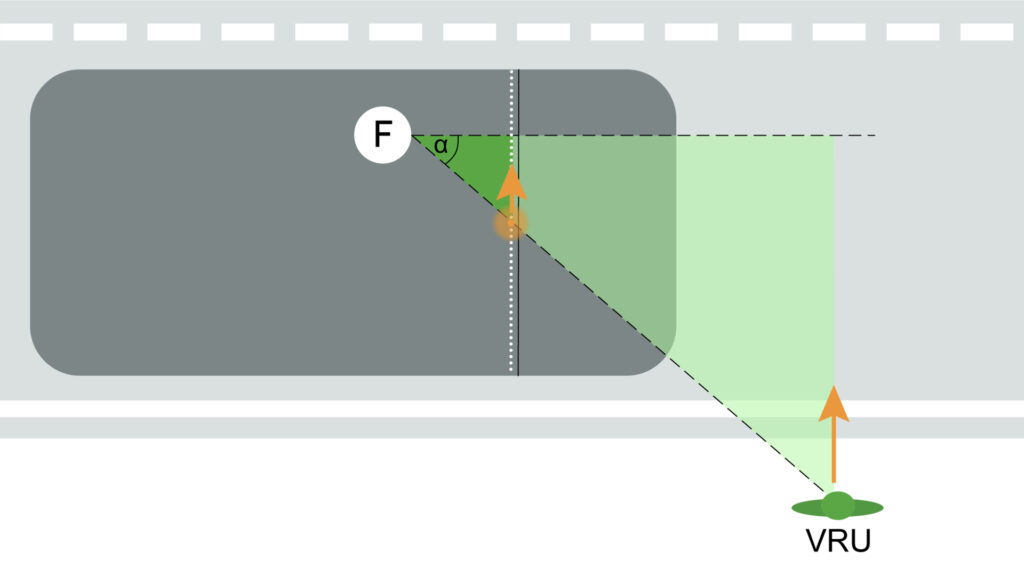
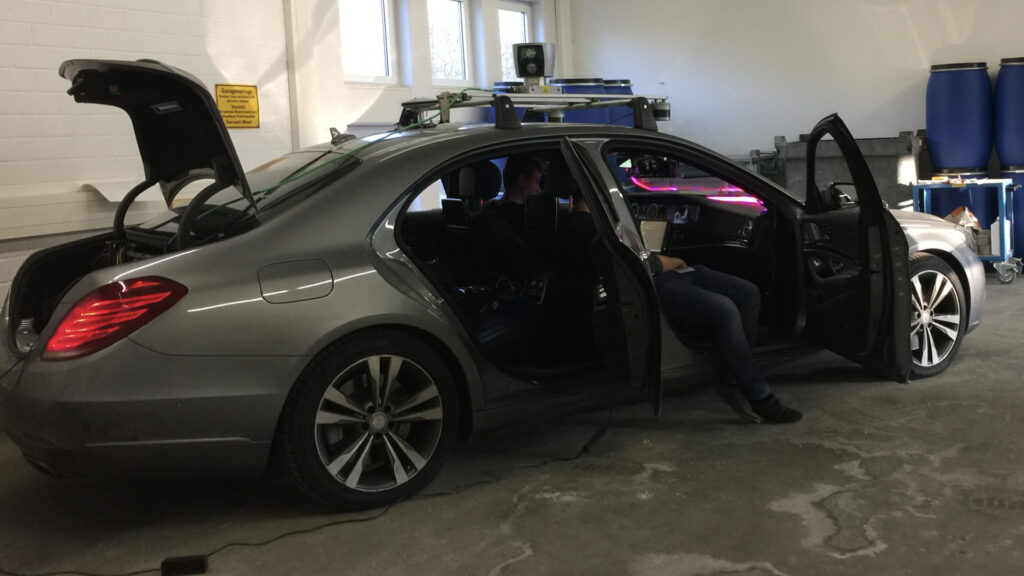
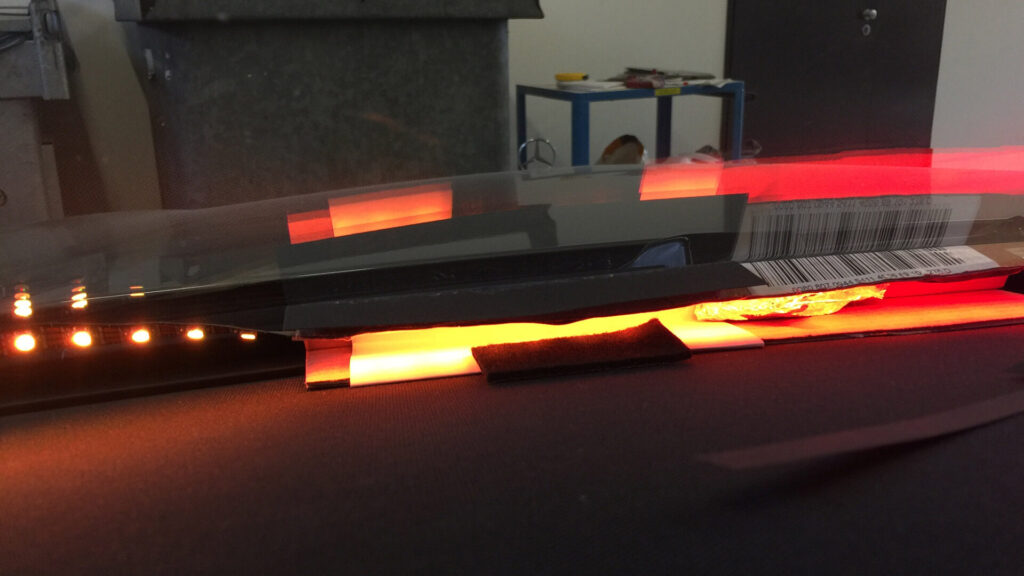
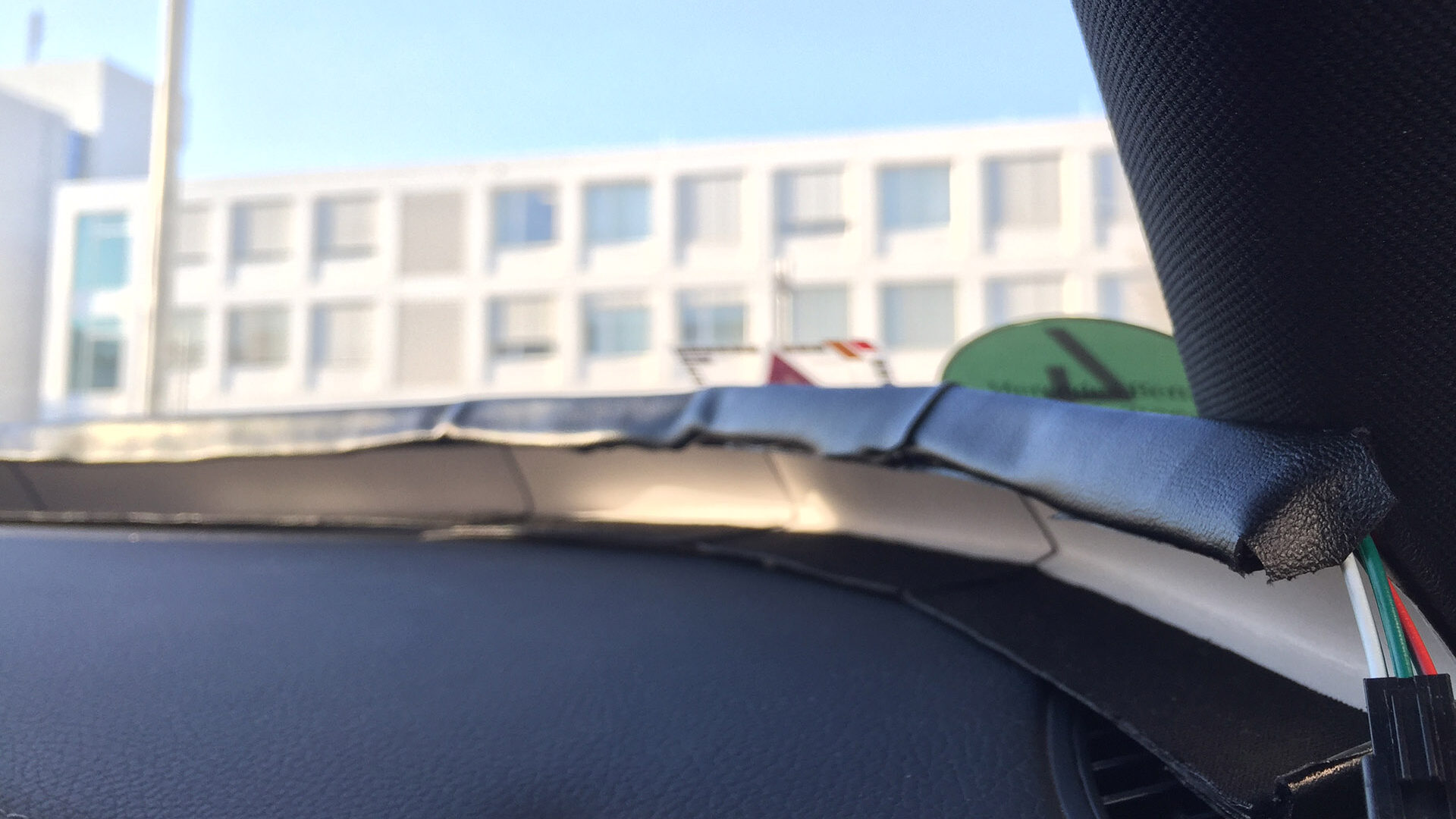
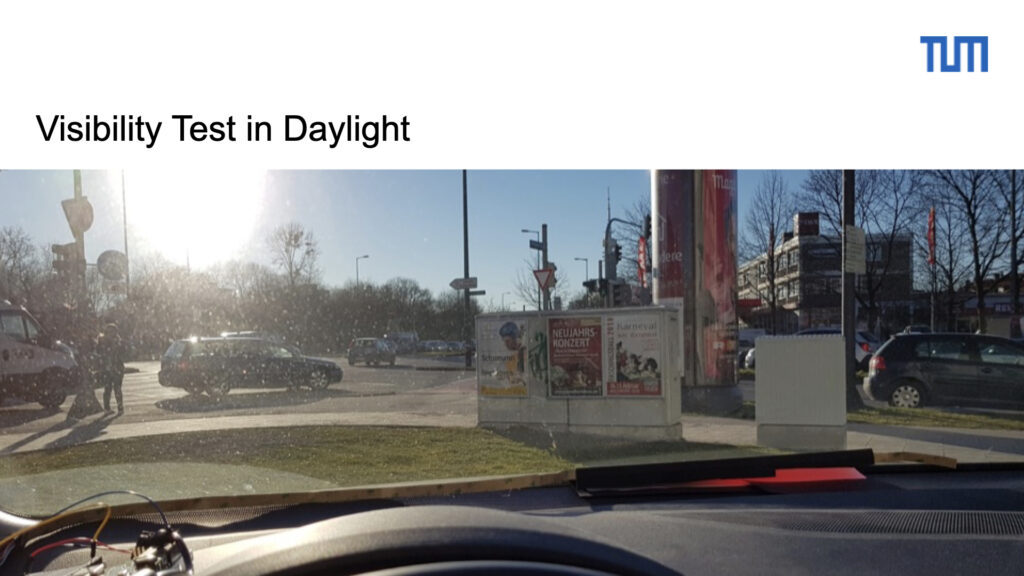
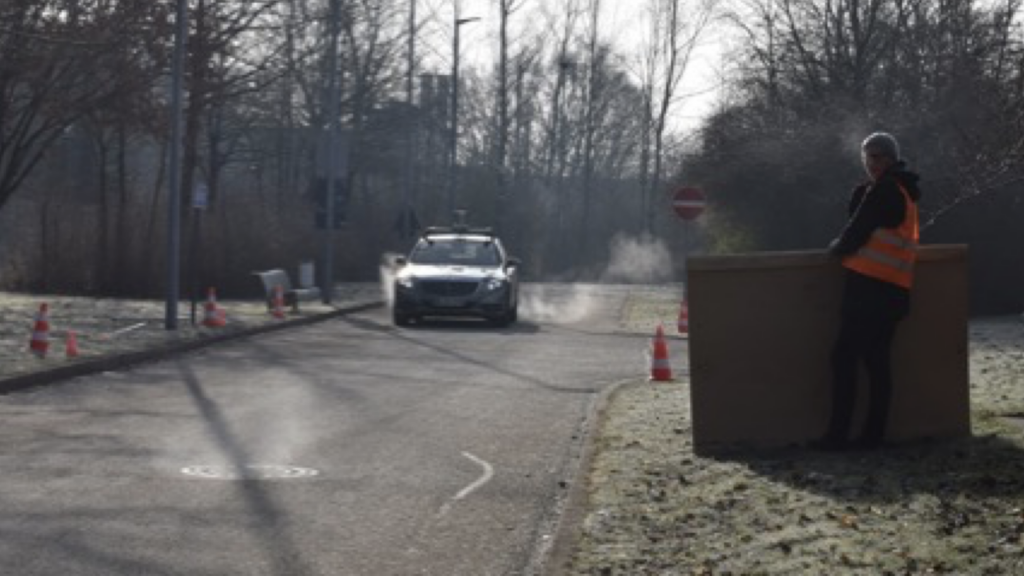
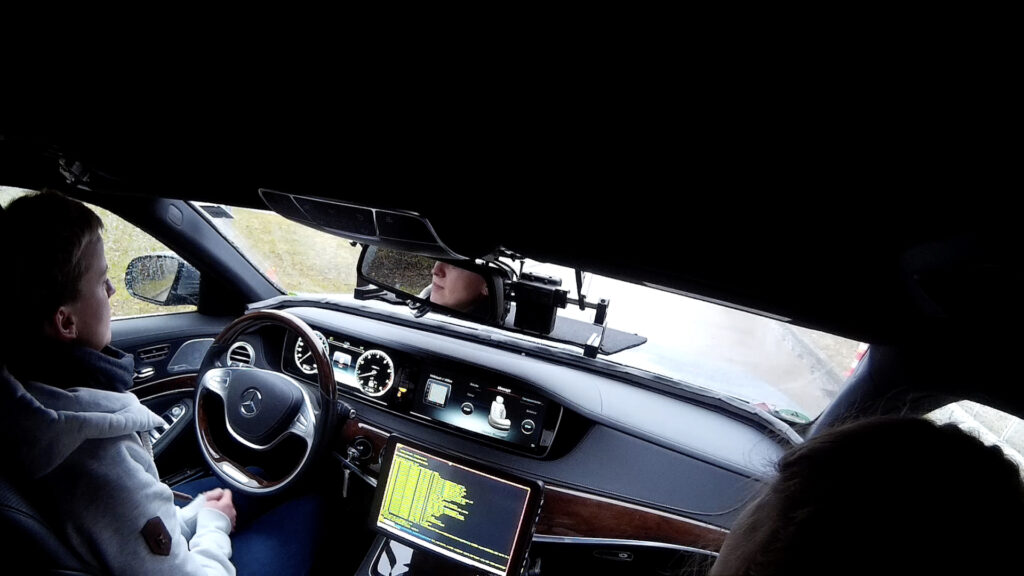
Evaluation
The developed HMI concept was tested and evaluated in a Mercedes Benz S-Class V-222 on the Daimler campus with twelve professional test drivers (Daimler employees) in February 2018. Each session took about 1 hour.
Evaluation criteria: acceptance, trust, usefulness / assessment of safety gain and distraction, comprehensibility and usability, design, appropriateness in the respective situations.
Test Scenarios
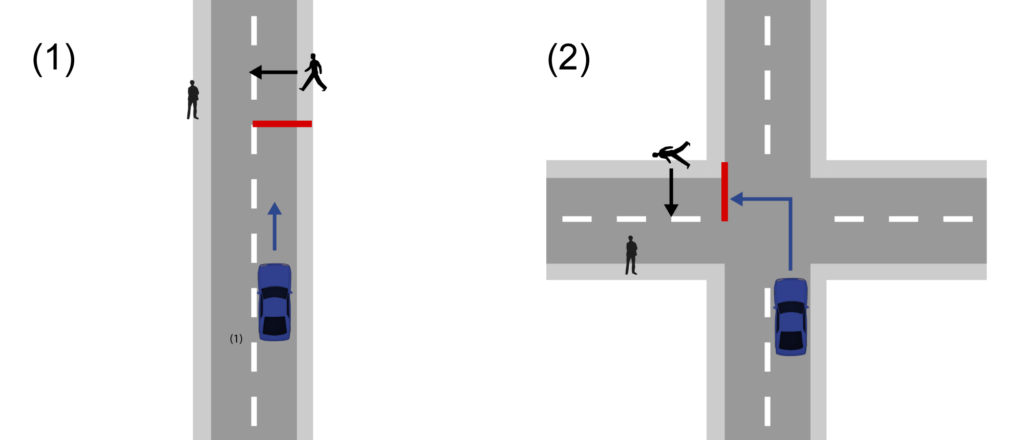
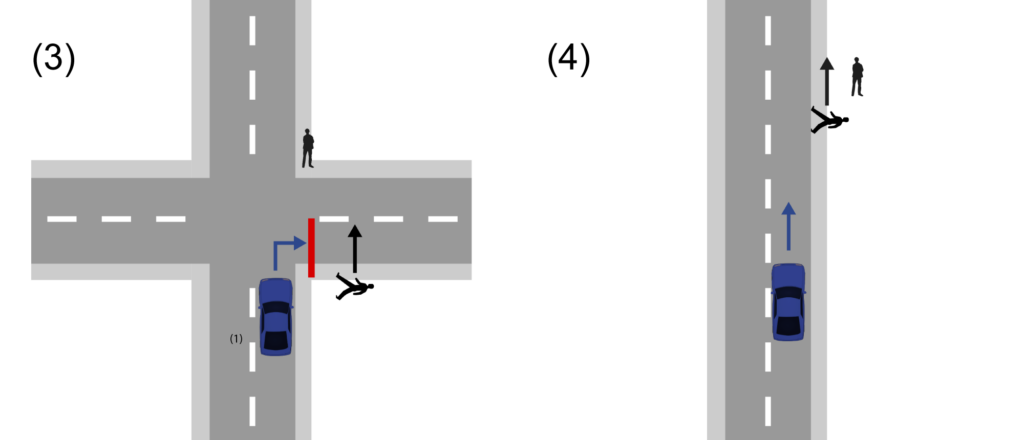
Sample
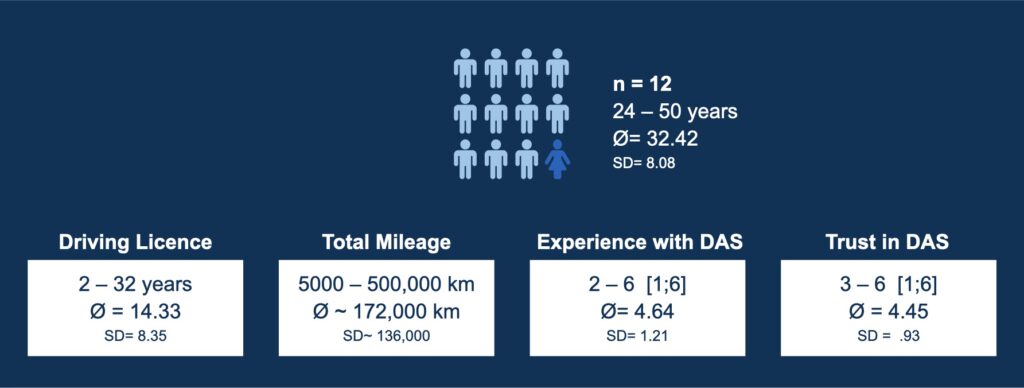
Results, Limitations, and Optimization Potential
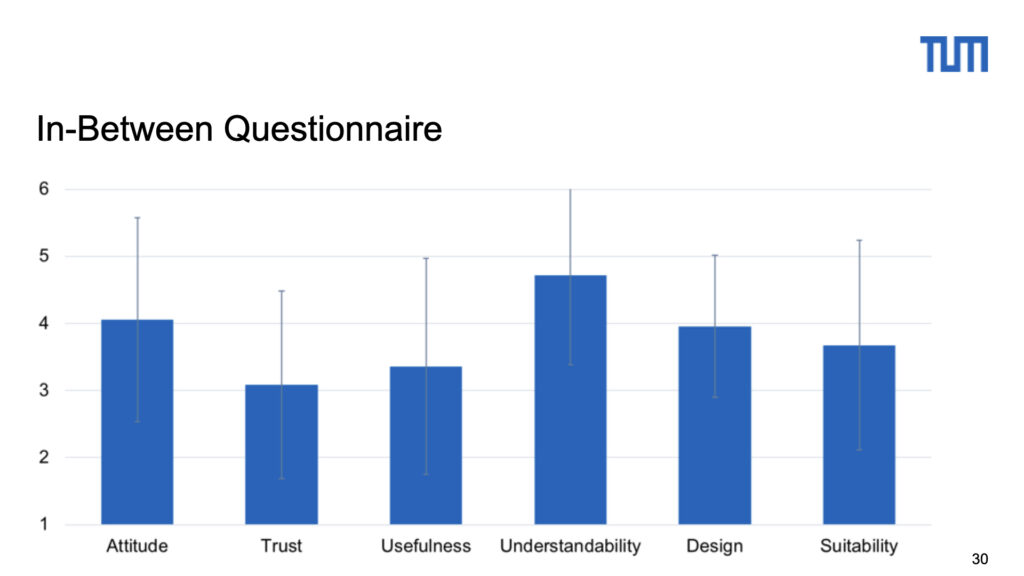
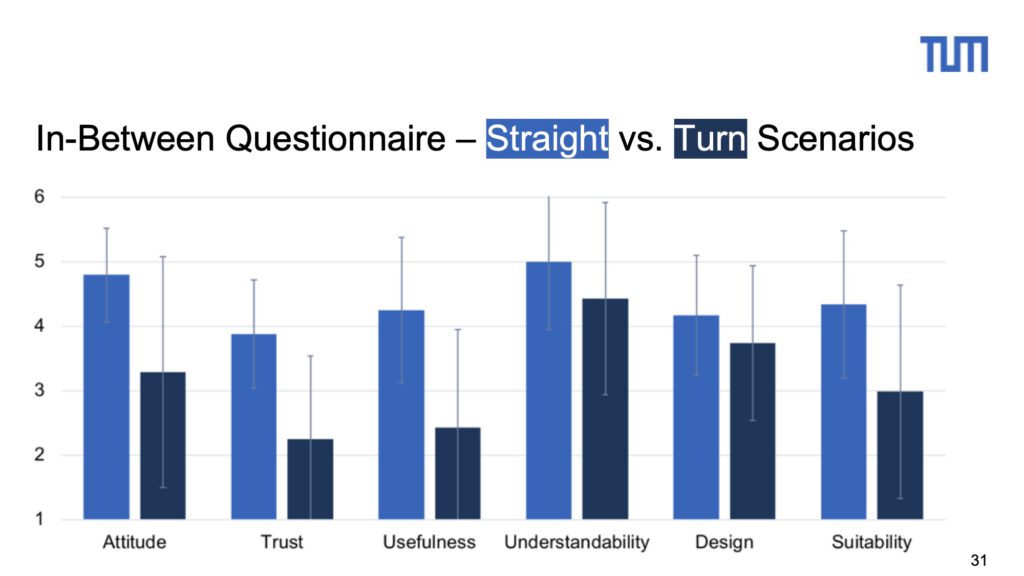
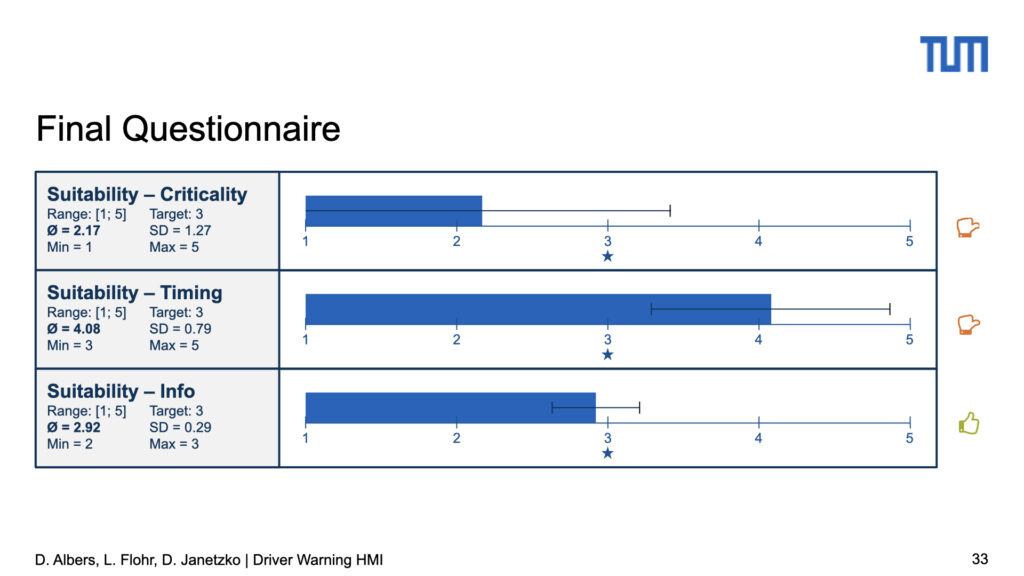
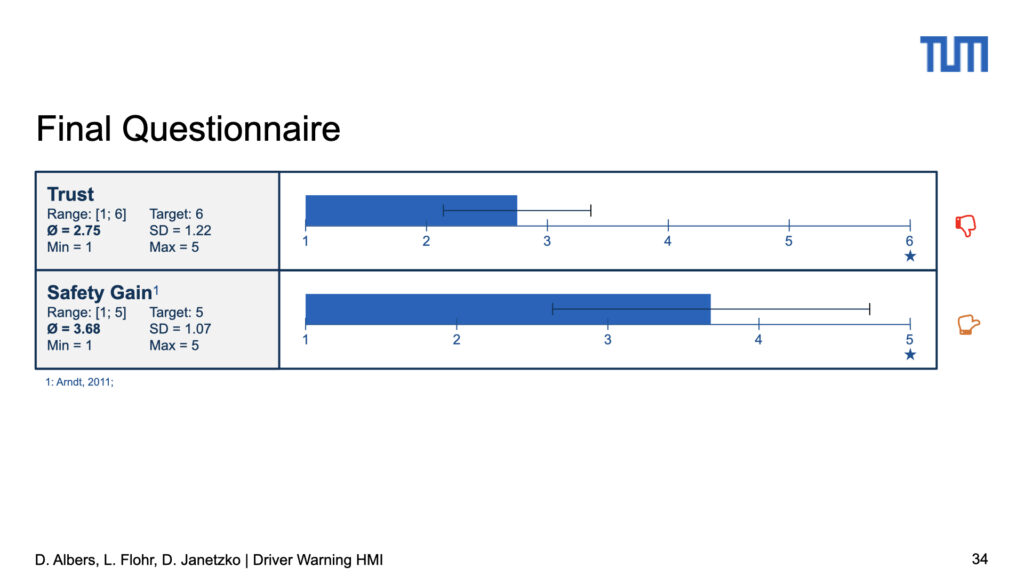
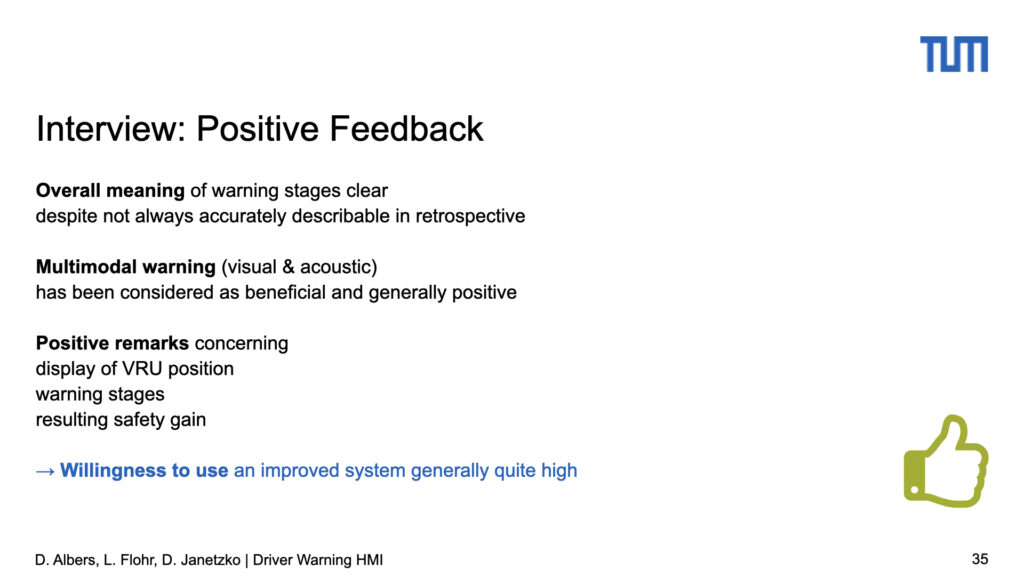
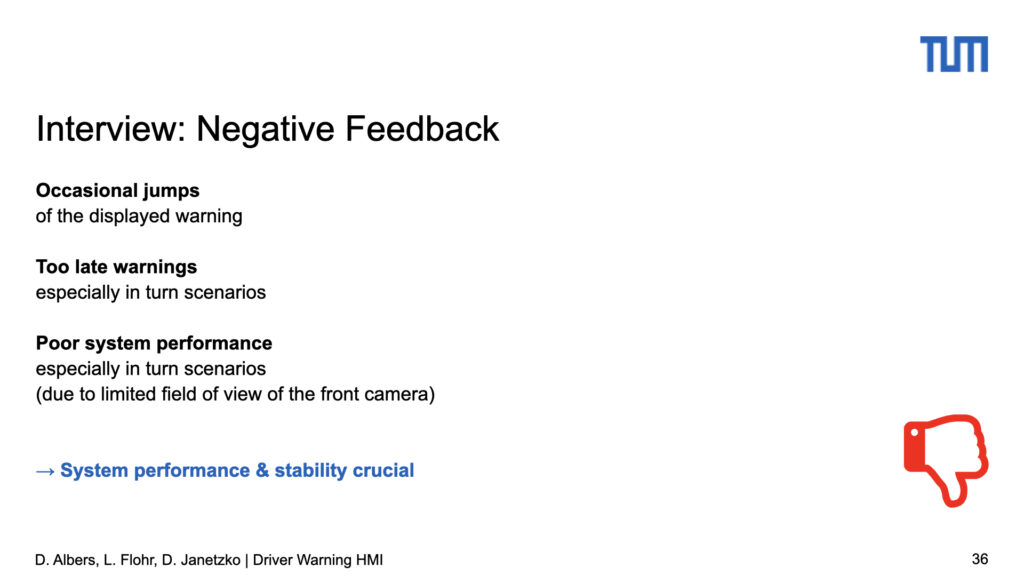
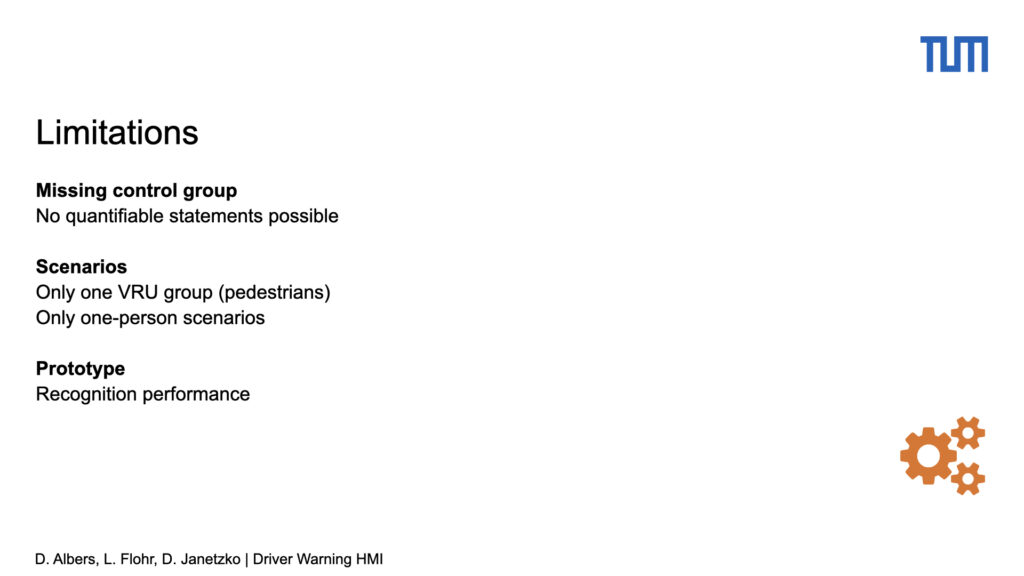
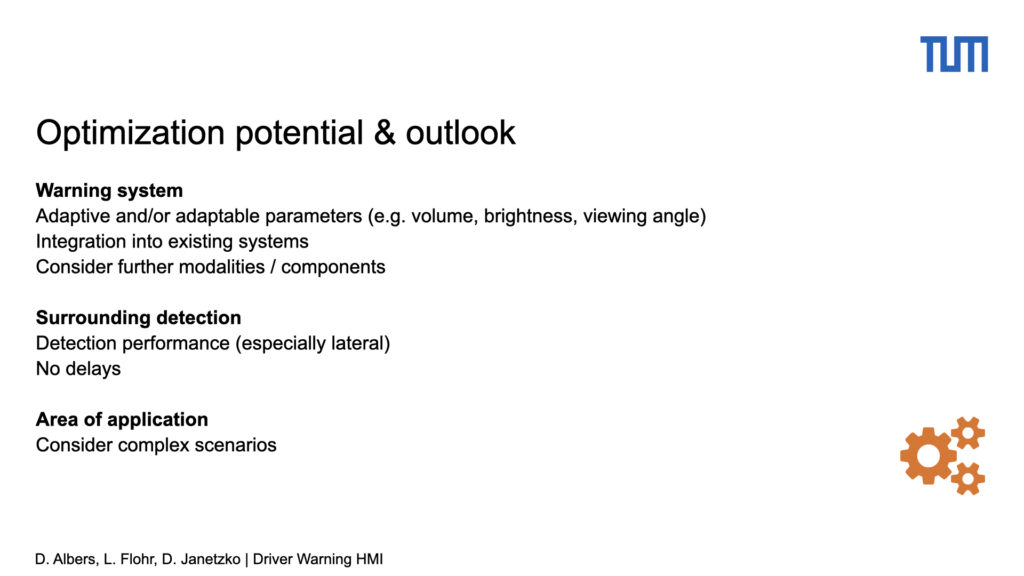
Project Management
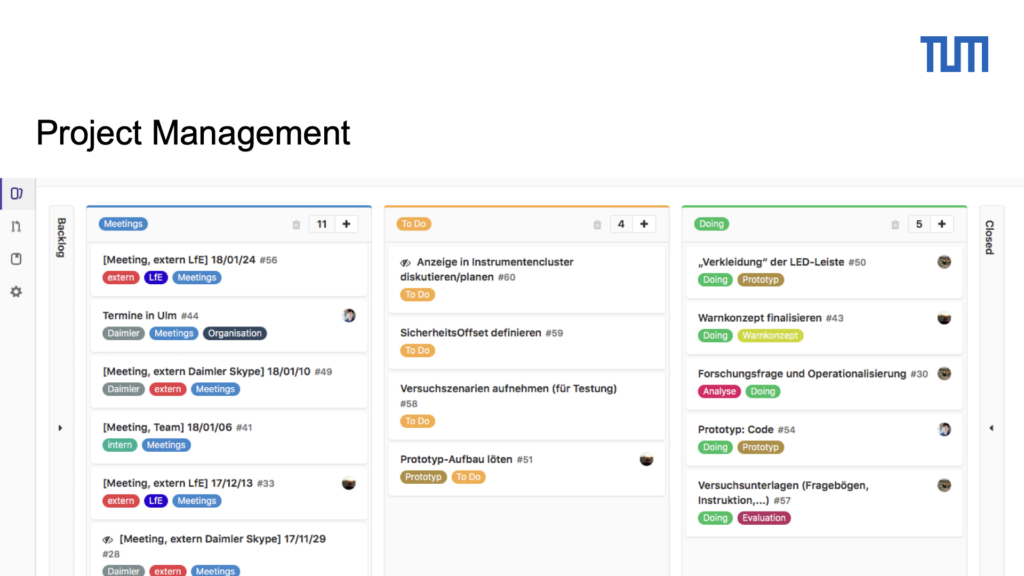
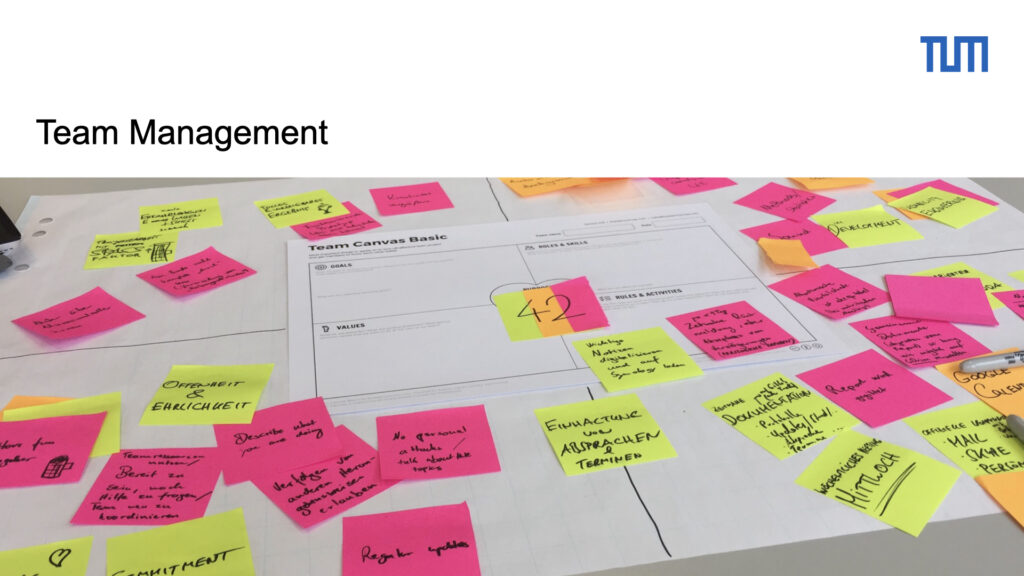
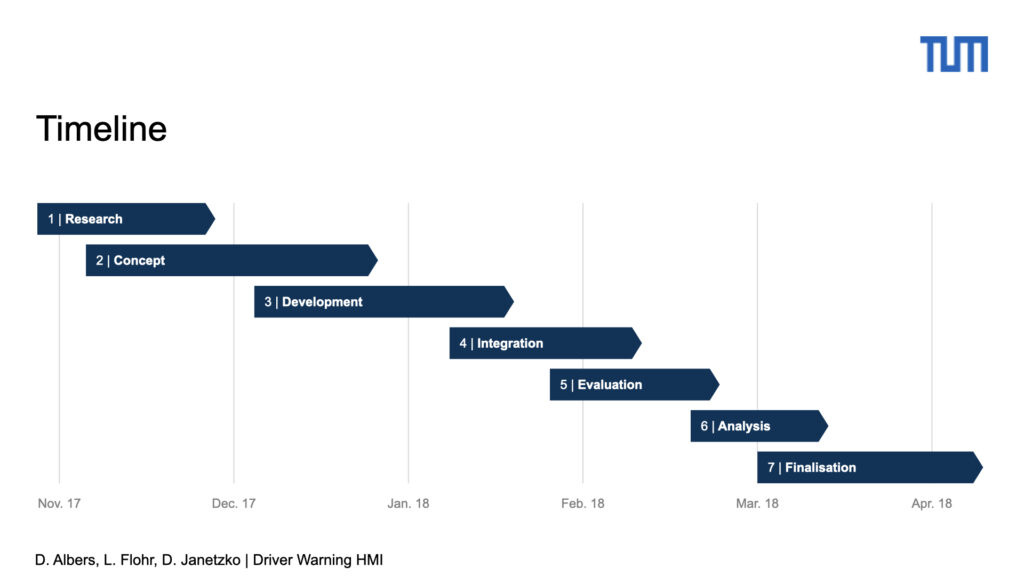
References
Arndt, S. (2011). Evaluierung der Akzeptanz von Fahrerassistenzsystemen. VS Verlag für Sozialwissenschaften.
Bainbridge, L. (1983). Ironies of automation. Automatica, 19(6), 775–779. doi:10.1016/0005-1098(83)90046-8
Bengler, K., Dietmayer, K., Färber, B., Maurer, M., Stiller, C. & Winner, H. (2014). Three Decades of Driver Assistance Systems Review and Future Perspectives. IEEE Intelligent Transportation Systems Magazine, 6(October), 6–22.
Bengler, K., Drüke, J., Hoffmann, S., Manstetten, D., & Neukum, A. (2018). UR:BAN Human Factors in Traffic. (K. Bengler, J. Drüke, S. Hoffmann, D. Manstetten, & A. Neukum, Hrsg.). Wiesbaden: Springer Fachmedien Wiesbaden. http://doi.org/10.1007/978-3-658-15418-9
Diederichs, F., Brouwer, N., Klöden, H., Zahn, P. & Schmitz, B. (2018). Application of a Driver Intention Recognition Algorithm on a Pedestrian Intention Recognition and Collision Avoidance System. In UR:BAN Human Factors in Traffic (S. 267–284). Wiesbaden: Springer Vieweg. doi:10.1007/978-3-658-15418-9{\_}14
Drüke, J., Semmler, C. & Bendewald, L. (2018). The “HMI tool kit” as a Strategy for the Systematic Derivation of User-Oriented HMI Concepts of Driver Assistance Systems in Urban Areas. In UR:BAN Human Factors in Traffic (Kap. 4, S. 53–74). Wiesbaden: Springer Vieweg. doi:10.1007/978-3-658-15418-9{\_}4
Götze, M., Bißbort, F., Petermann-Stock, I. & Bengler, K. (2014). A careful driver is one who looks in both directions when he passes a red light Increased demands in urban traffic. In Lecture Notes in Computer Science (including subseries Lecture Notes in Artificial Intelligence and Lecture Notes in Bioinformatics) (Bd. 8522 LNCS, PART 2, S. 229–240). doi:10.1007/978-3-319-07863-2{\_}23
Mahlke, S., Rösler, D., Seifert, K., Krems, J. F. & Thüring, M. (2007). Evaluation of six night vision enhancement systems. Qualitative and quantitative support for intelligent image processing. Human factors, 49 (3), 518–531. https://doi.org/10.1518/001872007X200148
Petermann-Stock, I., Werneke, J., (2014). Projekt: Mensch im Verkehr – 2. Meilensteinbericht (Q8) Teilprojekt: Stadtgerechte Mensch-Machine-Interaktion – MMI AP 2000: Entwurf; AP 3000: Test und Evaluation. UR:BAN. Wolfsburg
UR:BAN. (2016). UR:BAN Projekt : Mensch im Verkehr – 4. Meilensteinbericht (Q16) Teilprojekt: Stadtgerechte Mensch-Maschine-Interaktion – MMI AP 5000: Test und Evaluation (2. Phase). Wolfsburg.
Utesch Fabian. (2014, 28. April). Unscharfe Warnungen im Kraftfahrzeug. Eignet sich eine LED-Leiste als Anzeige für Fahrerassistenzsysteme? Dissertation. Technische Universität Carolo-Wilhelmina zu Braunschweig, Lüneburg.
Van Der Laan, J. D., Heino, A., & De Waard, D. (1997). A simple procedure for the assessment of acceptance of advanced transport telematics. Transportation Research Part C: Emerging Technologies, 5(1), 1-10.
Winkler, S., Powelleit, M., Kazazi, Juela, Vollrath, M., Krautter, W., Korthauer, A., . . . & Semmler, C. (2018). HMI Strategy – Warnings and Interventions. In UR:BAN Human Factors in Traffic (S. 75–102). Wiesbaden: Springer Vieweg.
World Health Organization. (2015). Global Status Report on Road Safety 2015. Genf. doi:10.1136/injuryprev-2013-040775
Metadata
Type
Project Team (TUM)
Advisors (TUM)
Project Partners (Daimler AG)
Institutions
Presentation
Report
Activities & Responsibilities
Project initiation & mangement
Research & contextual analysis
Conceptual design
Hardware prototyping
Software development
(Arduino, C++)
Evaluation
(expert-based user testing)
Data analysis